Machine Learning Methods for Virus–Host Protein–Protein Interaction Prediction
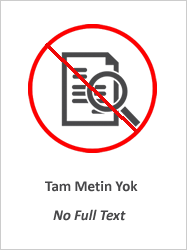
Tarih
2023Yazar
Karpuzcu, Betül AsiyeTürk, Erdem
İbrahim, Ahmad Hassan
Karabulut, Onur Can
Süzek, Barış Ethem
Üst veri
Tüm öğe kaydını gösterKünye
Karpuzcu BA, Türk E, Ibrahim AH, Karabulut OC, Süzek BE. Machine Learning Methods for Virus-Host Protein-Protein Interaction Prediction. Methods Mol Biol. 2023;2690:401-417. doi: 10.1007/978-1-0716-3327-4_31. PMID: 37450162.Özet
The attachment of a virion to a respective cellular receptor on the host organism occurring through the virus–host protein–protein interactions (PPIs) is a decisive step for viral pathogenicity and infectivity. Therefore, a vast number of wet-lab experimental techniques are used to study virus–host PPIs. Taking the great number and enormous variety of virus–host PPIs and the cost as well as labor of laboratory work, however, computational approaches toward analyzing the available interaction data and predicting previously unidentified interactions have been on the rise. Among them, machine-learning-based models are getting increasingly more attention with a great body of resources and tools proposed recently. In this chapter, we first provide the methodology with major steps toward the development of a virus–host PPI prediction tool. Next, we discuss the challenges involved and evaluate several existing machine-learning-based virus–host PPI prediction tools. Finally, we describe our experience with several ensemble techniques as utilized on available prediction results retrieved from individual PPI prediction tools. Overall, based on our experience, we recognize there is still room for the development of new individual and/or ensemble virus–host PPI prediction tools that leverage existing tools.