Multiple Brain Tumor Classification with Dense CNN Architecture Using Brain MRI Images
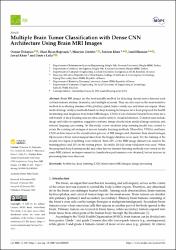
Göster/Aç
Tarih
2023Yazar
Özkaraca, OsmanBağrıaçık, Okan İhsan
Gürüler, Hüseyin
Khan, Faheem
Hussain, Jamil
Khan, Jawad
Laila, Umm e
Üst veri
Tüm öğe kaydını gösterKünye
Özkaraca, O.; Bağrıaçık, O. ̇I.; Gürüler, H.; Khan, F.; Hussain, J.; Khan, J.; Laila, U.e. Multiple Brain Tumor Classification with Dense CNN Architecture Using Brain MRI Images. Life 2023, 13, 349. https://doi.org/10.3390/life13020349Özet
Brain MR images are the most suitable method for detecting chronic nerve diseases such as brain tumors, strokes, dementia, and multiple sclerosis. They are also used as the most sensitive method in evaluating diseases of the pituitary gland, brain vessels, eye, and inner ear organs. Many medical image analysis methods based on deep learning techniques have been proposed for health monitoring and diagnosis from brain MRI images. CNNs (Convolutional Neural Networks) are a sub-branch of deep learning and are often used to analyze visual information. Common uses include image and video recognition, suggestive systems, image classification, medical image analysis, and natural language processing. In this study, a new modular deep learning model was created to retain the existing advantages of known transfer learning methods (DenseNet, VGG16, and basic CNN architectures) in the classification process of MR images and eliminate their disadvantages. Open-source brain tumor images taken from the Kaggle database were used. For the training of the model, two types of splitting were utilized. First, 80% of the MRI image dataset was used in the training phase and 20% in the testing phase. Secondly, 10-fold cross-validation was used. When the proposed deep learning model and other known transfer learning methods were tested on the same MRI dataset, an improvement in classification performance was obtained, but an increase in processing time was observed.